The Data Is in: Deciding When to Automate Screening in Your SLR
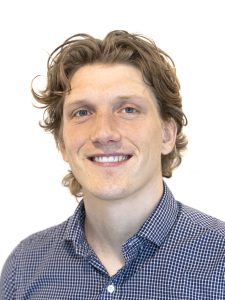
Karl Holub
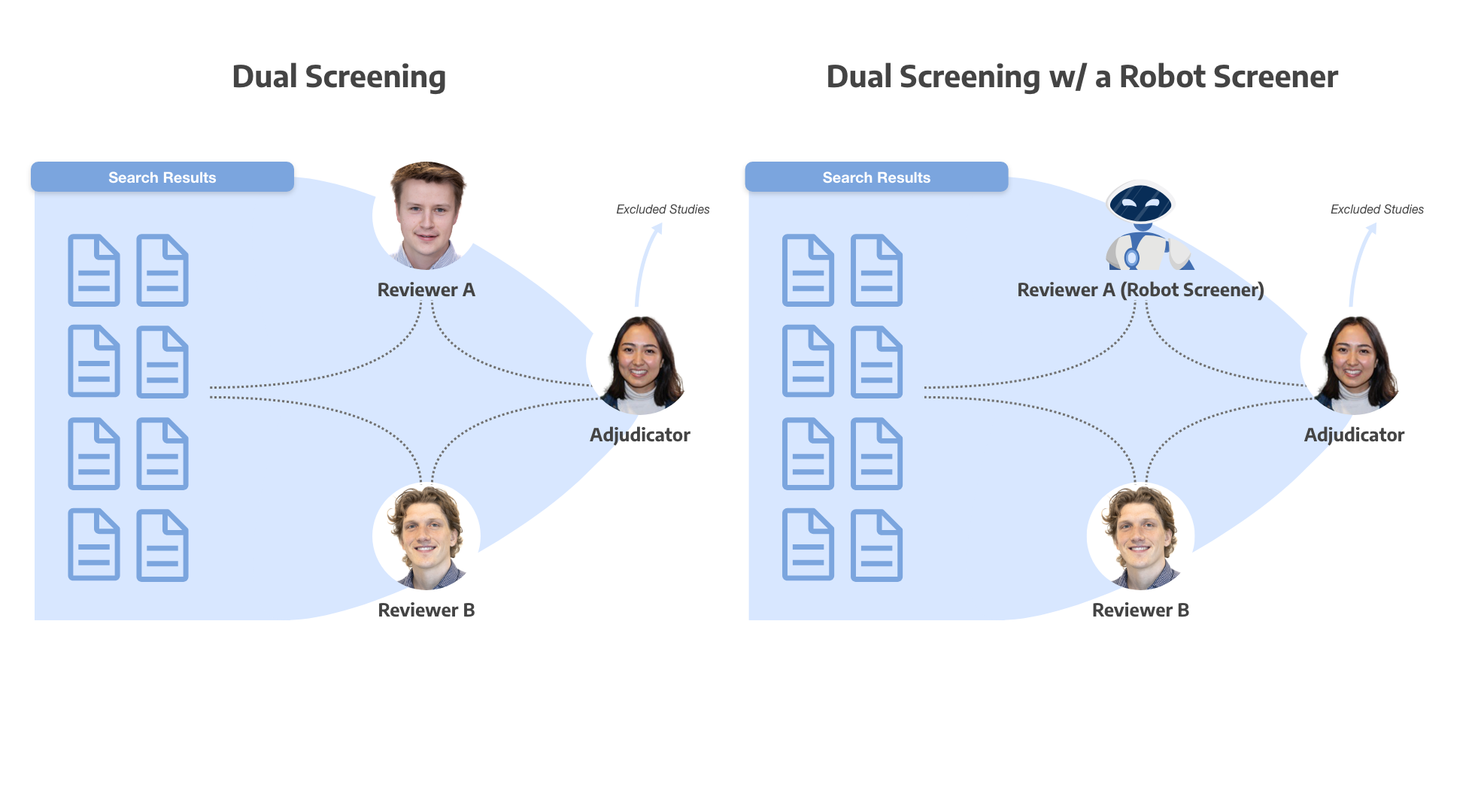
When conducting a Systematic Literature Review (SLR), researchers cast a wide net searching for potentially relevant studies and evidence. In many SLR use cases (e.g. regulatory reporting, health technology assessment, scholarly publication), not identifying includable evidence can lead to lower confidence or incorrect findings. However, by casting a wide net to ensure such evidence is not missed, the review’s Search will also bring back irrelevant evidence. Screening is the step in an SLR that cleans up this state affairs, identifying which evidence is relevant for inclusion in the synthesis–but since high-quality Screening usually involves two reviewers checking every study, it can be an extremely time-intensive process.
Enter Robot Screener, an AutoLit product offering that augments screening by replacing one of those reviewers. It’s available to any nest (including free tier!) configured for dual screening, whether standard or two pass. Why might you use Robot Screener in a review?
- You want to achieve a higher level of rigor in your review than single screening would allow
- You need to deliver a review with dual screening, but are subject to time or personnel constraints
Let’s explore some details that will help you decide if Robot Screener is right for your next review!
Metrics to consider in Screening
When choosing your screening methodology, two key measures to consider are:
- Recall: How much of the evidence relevant to your research question is included in your review? It’s a reality that not all relevant evidence will be identified by screeners, often for good reason! For example, there may be poor information density in abstracts or differing interpretations of the protocol and research question. Recall is the percentage of records included in a review amongst all records that should be included in the review.
- For a point of reference, we’ve estimated that a single human screener’s recall falls between 85-90% on an “average” review.
- Time: How long does the screening process take in person-hours? This equates to money and publication delay; shorter is better, provided recall remains acceptable.
- Haddaway 2018 estimates that screening consumes 20% of the total time to produce a published systematic review (equating to 33 days, where the average review size was around 8,000 records).
These outcomes form a trade off, as we’ll see with Robot Screener and even human workflows.
Robot & Recall
In screening, there are two mistakes a reviewer (human or robot) can make:
- Include a record that should be excluded in actuality (false positive)
- The consequence of this mistake is lost time- it is quite likely that the improper inclusion will be discovered & excluded later in extraction, appraisal, or reporting.
- Exclude a record that should be included (false negative)
- With the consequence that your review will be missing evidence.
- With the consequence that your review will be missing evidence.
At Nested Knowledge, we consider false negatives to be the more costly mistake & preferentially optimize against it.
The 85% human-reviewer recall reported above derives from a study of dual screening reviewers against adjudicated decisions, across 100s of nests made available in an internal study. If we make the simplifying assumption that reviewers make screening mistakes independent of one another and that the adjudicator will automatically accept any agreement between reviewers, it means that 15% * 15% = 2.25% of records that should be included in the review were not. i.e. the combined recall approaches 98%. To put this in real terms, in a review where 100 studies should have been included, we’d expect only 98 of them to be included by dual screening.
What happens when we substitute Robot Screener for a human screener? Internal study has shown that Robot Screener sports a recall of 80% in practice (an important distinction, based on how and when users choose to enable it). To carry out the same calculations, with one human screener and Robot Screener, we’d expect 15% * 20% = 3% of records that should be included in the review to be excluded (97% recall).
This implies that enabling Robot Screener would have the following outcomes:
- Coming from a dual screening workflow: 1 in 100 studies that should have been included in your review won’t be
- Coming from a single screening workflow: 12 in 100 studies that would have been improperly excluded will now be included
Key Takeaways
- A single human screener’s recall falls between 85-90% on an “average” review.
- Screening consumes 20% of the total time to produce a published systematic review.
- While two human reviewers will perform better than human and a robot…the difference in average recall is around 1%.
- Using a robot in the place of one human reviewer can result in a time savings of up to 46% for standard dual screening.
- Switching from single screening mode to dual screening with a robot can increase the human reviewers recall by up to 15%, and decrease time spent by 15%.
Robot & Time
First, let’s figure out how long a typical human-only screening process takes. If we assume an adjudicator auto-adjudicates any reviewer agreement and only mediates disagreements, and that every reviewer requires the same amount of time to screen a record, the total time spent screening is:
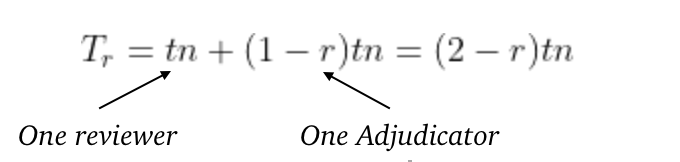
Where
- t = time to screen a record
- n = number of records screened
- h = human-human screening agreement rate
Next, we’ll analyze what happens when we substitute the Robot Screener for a human reviewer, ignoring the time needed to train the screening modal (which is consequential in small reviews). The total time spent screening is:

Where r = the human-robot screening agreement rate.
The ratio of time for robot augmented screening to human-only screening is:

Substituting values empirically derived from a sampling of 30 projects, h = .85 and r = .84:

This implies a time savings of 46% for standard dual screening. If the nest is configured for two pass screening (where the Robot Screener only weighs in on abstracts), we’d do well to cut this number at least in half (accounting for a doubling of time full text screening).
Bottom Line
If you’re coming from a dual screening workflow, Robot Screener could save 20-45% of your typical screening time, at an expected cost of 1% recall.
If you’re coming from a single screening workflow, dual screening with Robot Screener could increase your review’s recall 10-15%, at an approximate 15% screening time increase.
We think those tradeoffs are favorable for a large number of use cases—think about Robot Screener next time you’re building a nest. By replacing one of two dual Screeners, and having every single disagreement checked by a researcher, you are setting up your workflow to ensure human oversight of every AI decision. Final screening decisions should be in the hands of you and your team, under the general AI philosophy that human oversight is necessary for every final decision in the SLR process. AI should augment and provide both information and efficiency, but given the importance of comprehensiveness and accuracy to review findings, Robot Screener should not be employed in an SLR as a stand-alone screener.
Learn More
- Robot Screener Documentation: https://wiki.nested-knowledge.com/doku.php?id=wiki:autolit:screening:robot
- Details on the screening model powering Robot Screener and results from our internal studies: https://wiki.nested-knowledge.com/doku.php?id=wiki:autolit:screening:inclusionpredictionmodel
- Our CDO’s proudest video production: https://www.youtube.com/watch?v=aBCL3FgrnqA
A blog about systematic literature reviews?
Yep, you read that right. We started making software for conducting systematic reviews because we like doing systematic reviews. And we bet you do too.
If you do, check out this featured post and come back often! We post all the time about best practices, new software features, and upcoming collaborations (that you can join!).
Better yet, subscribe to our blog, and get each new post straight to your inbox.
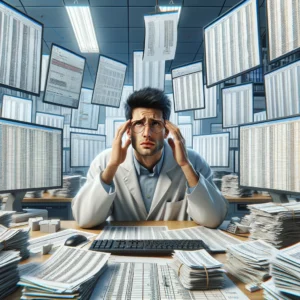
Feature Comparison for Systematic Literature Review: Nested Knowledge vs Spreadsheets
In the realm of conducting systematic literature reviews (SLRs), the question invariably arises: how do these new technologies compare to the trusty spreadsheet? Spreadsheets offer
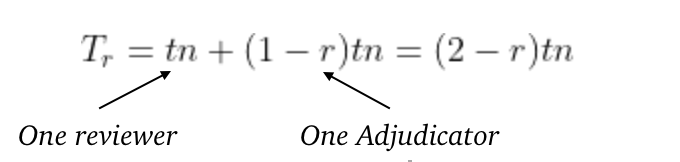